17: Pandas (the Basics) and Titanic Analysis#
import numpy as np
import matplotlib.pyplot as plt
import pandas as pd
1. Load your data into a Pandas DataFrame#
The most common options:
read_csv
read_excel
data = pd.read_excel('../data/titanic3 (3).xls')
data.shape
data.head()
pclass | survived | name | sex | age | sibsp | parch | ticket | fare | cabin | embarked | boat | body | home.dest | |
---|---|---|---|---|---|---|---|---|---|---|---|---|---|---|
0 | 1 | 1 | Allen, Miss. Elisabeth Walton | female | 29.0000 | 0 | 0 | 24160 | 211.3375 | B5 | S | 2 | NaN | St Louis, MO |
1 | 1 | 1 | Allison, Master. Hudson Trevor | male | 0.9167 | 1 | 2 | 113781 | 151.5500 | C22 C26 | S | 11 | NaN | Montreal, PQ / Chesterville, ON |
2 | 1 | 0 | Allison, Miss. Helen Loraine | female | 2.0000 | 1 | 2 | 113781 | 151.5500 | C22 C26 | S | NaN | NaN | Montreal, PQ / Chesterville, ON |
3 | 1 | 0 | Allison, Mr. Hudson Joshua Creighton | male | 30.0000 | 1 | 2 | 113781 | 151.5500 | C22 C26 | S | NaN | 135.0 | Montreal, PQ / Chesterville, ON |
4 | 1 | 0 | Allison, Mrs. Hudson J C (Bessie Waldo Daniels) | female | 25.0000 | 1 | 2 | 113781 | 151.5500 | C22 C26 | S | NaN | NaN | Montreal, PQ / Chesterville, ON |
data.describe()
pclass | survived | age | sibsp | parch | fare | body | |
---|---|---|---|---|---|---|---|
count | 1309.000000 | 1309.000000 | 1046.000000 | 1309.000000 | 1309.000000 | 1308.000000 | 121.000000 |
mean | 2.294882 | 0.381971 | 29.881135 | 0.498854 | 0.385027 | 33.295479 | 160.809917 |
std | 0.837836 | 0.486055 | 14.413500 | 1.041658 | 0.865560 | 51.758668 | 97.696922 |
min | 1.000000 | 0.000000 | 0.166700 | 0.000000 | 0.000000 | 0.000000 | 1.000000 |
25% | 2.000000 | 0.000000 | 21.000000 | 0.000000 | 0.000000 | 7.895800 | 72.000000 |
50% | 3.000000 | 0.000000 | 28.000000 | 0.000000 | 0.000000 | 14.454200 | 155.000000 |
75% | 3.000000 | 1.000000 | 39.000000 | 1.000000 | 0.000000 | 31.275000 | 256.000000 |
max | 3.000000 | 1.000000 | 80.000000 | 8.000000 | 9.000000 | 512.329200 | 328.000000 |
2. Clean up your dataset with drop(), dropna() and fillna()#
data = data.drop(['name', 'sibsp', 'parch', 'ticket', 'fare', 'cabin', 'embarked', 'boat', 'body', 'home.dest'], axis=1)
data = data.dropna(axis=0)
data.shape
(1046, 4)
data['age'].hist()
<Axes: >
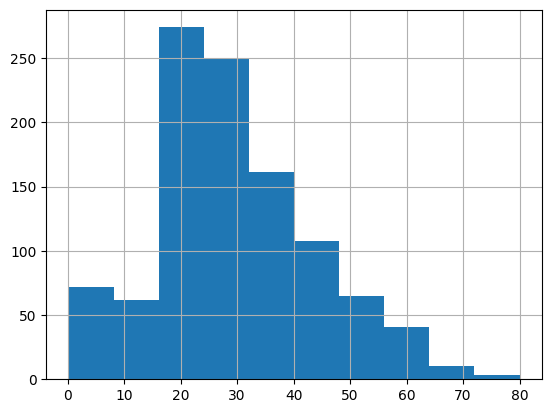
3. Groupby() and value_counts()#
data.groupby(['sex']).mean()
pclass | survived | age | |
---|---|---|---|
sex | |||
female | 2.048969 | 0.752577 | 28.687071 |
male | 2.300912 | 0.205167 | 30.585233 |
data.groupby(['sex', 'pclass']).mean()
survived | age | ||
---|---|---|---|
sex | pclass | ||
female | 1 | 0.962406 | 37.037594 |
2 | 0.893204 | 27.499191 | |
3 | 0.473684 | 22.185307 | |
male | 1 | 0.350993 | 41.029250 |
2 | 0.145570 | 30.815401 | |
3 | 0.169054 | 25.962273 |
data['pclass'].value_counts()
3 501
1 284
2 261
Name: pclass, dtype: int64
data[data['age'] < 18]['pclass'].value_counts()
3 106
2 33
1 15
Name: pclass, dtype: int64
4. Exercice#
Créer des catégories d’ages avec la fonction map() de pandas
Créer des catégories de genres avec cat.codes
Solution#
Show code cell content
def category_ages(age):
if age <= 20:
return '<20 ans'
elif (age > 20) & (age <= 30):
return '20-30 ans'
elif (age > 30) & (age <= 40):
return '30-40 ans'
else:
return '+40 ans'
Show code cell content
data['age'] = data['age'].map(category_ages)
Show code cell content
data['sex'].astype('category').cat.codes
0 0
1 1
2 0
3 1
4 0
..
1301 1
1304 0
1306 1
1307 1
1308 1
Length: 1046, dtype: int8